Statistics are, as the Merriam-Webster dictionary points out, “a collection of quantitative data”. Pure and simple. What is not that simple is how we use this data or how we make sense of it.
Fortunately, we live in a time and age where we have at the disposal of our fingertips incredible sources (in quantity and quality) of valuable information – including all kinds of stats – about baseball. We can check how good the spin rate of Justin Verlander’s Fastball is (fairly good) or how Matthew Boyd’s 2019 ERA is deceptive according to advanced stats like xFIP and SIERA, so, even after being roughed up the first few starts he’s made this season, he should be better this year.
I like spin rates and SIERA and a ton of other advanced stats, and the information we can get from them surpasses most of the time what we can analyze from speed (mph) or ERA, to name a couple of traditional stats. Nevertheless, I also like to try to simplify things but still be able to obtain powerful insights to make educated decisions.
NOTHING GETS SIMPLER THAN BALLS AND STRIKES.
I mean, we know them so well that even before the ubiquitous virtual strike zones we see nowadays, we could instantly start shouting to the umpire when we thought he was missing the calls. And we’ll do it forever because we KNOW balls and strikes. We know that more strikes than balls will always be good and pitchers that can do that are usually bound to have more success.
K%-BB% and (k-bb)/ip (let’s call them the K-Bs stats) are a couple of stats that exist just because of balls and strikes. They summarize in a straightforward way the achievement a pitcher has over what are the two principal outcomes that he can directly influence the most during an outing: strikeouts and walks.
Strikeout rate (K%) and walk rate (BB%) are calculations on how often a pitcher strikes out or walks batters per plate appearance (PA). You can calculate them by dividing the total number of strikeouts or walks a pitcher issued between the plate appearances batters got against him during a period of time (a week, month, season, etc.). Then, for the purpose of getting K%-BB%, you just subtract them and that’s it.
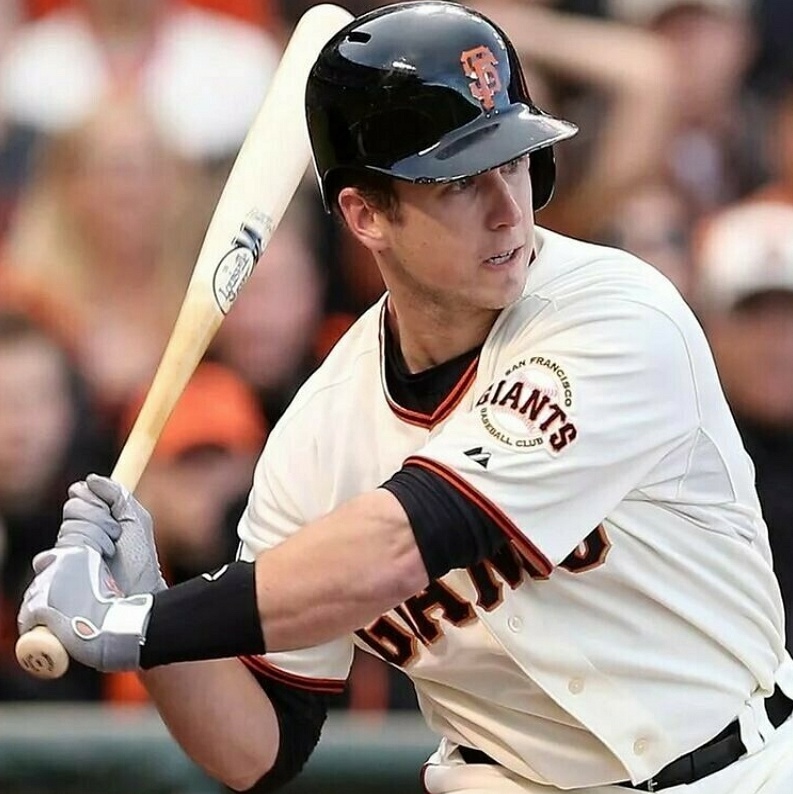
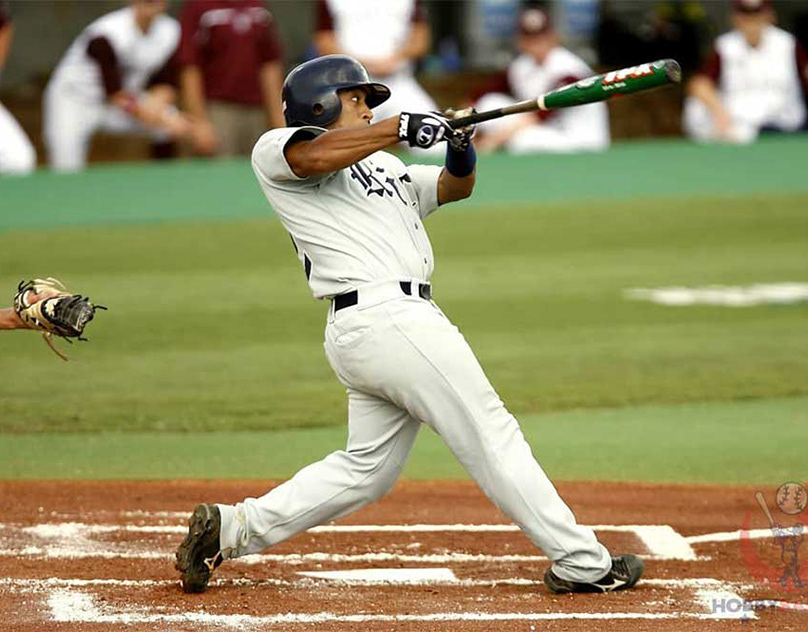
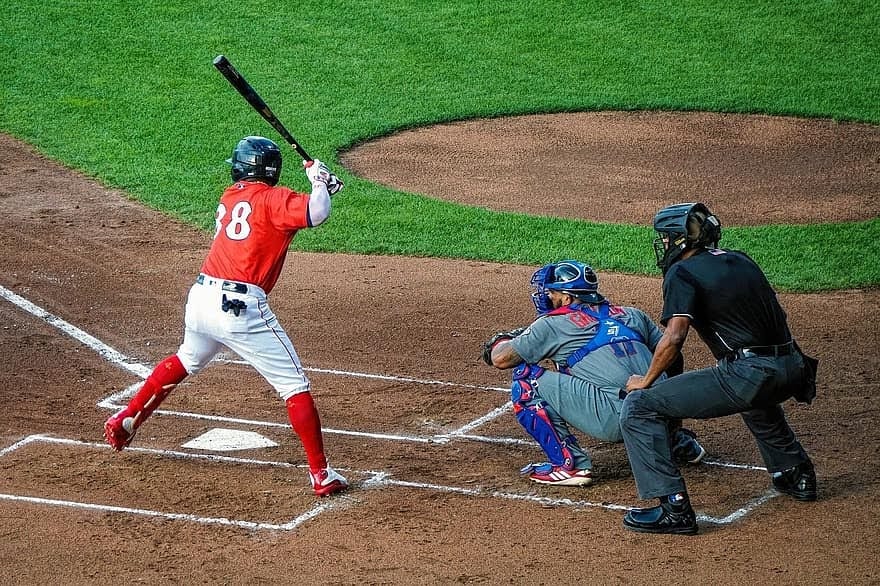
(k-bb)/ip goes similarly but you subtract strikeouts minus base on balls first and then divide the result between innings pitched. The reason for this (and comparably for dividing between plate appearances in K%-BB%) is to obtain ratios or proportions that allow us to compare pitchers who have faced vastly different quantities of batters.
If interested, you can find some more info on these stats here. By the way, Bill James was not a fan of them about 10 years ago, but Tom Tango was. What intrigues me the most about these stats is if they could be used to anticipate how a pitcher will fare afterward, their predictability; that’s why I decided to do some calculations to bring light on that. Some folks have done some writing about this but their conclusions are not that clear to me, so, I wanted to do research of my own.
First, I pulled data for pitchers during the 2019 season: main interest is on K%-BB% and (k-bb)/ip and also on ERA and some ERA estimators like xFIP, SIERA and of course our homegrown Predictive Classified Run Average, pCRA, which produces better estimates than SIERA. Note that I am including as a consistency check another estimator called CSW, which in 2019 was already proven to have a great correlation with SIERA and could be especially useful as a simple predictive stat, too.
Pitchers were restricted to those who had at least 23 or more games started as I wanted players with a lot of innings pitched, for a bigger and stabler sample.